-
Notifications
You must be signed in to change notification settings - Fork 1
/
Copy path7-tidy-text.html
547 lines (393 loc) · 16.9 KB
/
7-tidy-text.html
1
2
3
4
5
6
7
8
9
10
11
12
13
14
15
16
17
18
19
20
21
22
23
24
25
26
27
28
29
30
31
32
33
34
35
36
37
38
39
40
41
42
43
44
45
46
47
48
49
50
51
52
53
54
55
56
57
58
59
60
61
62
63
64
65
66
67
68
69
70
71
72
73
74
75
76
77
78
79
80
81
82
83
84
85
86
87
88
89
90
91
92
93
94
95
96
97
98
99
100
101
102
103
104
105
106
107
108
109
110
111
112
113
114
115
116
117
118
119
120
121
122
123
124
125
126
127
128
129
130
131
132
133
134
135
136
137
138
139
140
141
142
143
144
145
146
147
148
149
150
151
152
153
154
155
156
157
158
159
160
161
162
163
164
165
166
167
168
169
170
171
172
173
174
175
176
177
178
179
180
181
182
183
184
185
186
187
188
189
190
191
192
193
194
195
196
197
198
199
200
201
202
203
204
205
206
207
208
209
210
211
212
213
214
215
216
217
218
219
220
221
222
223
224
225
226
227
228
229
230
231
232
233
234
235
236
237
238
239
240
241
242
243
244
245
246
247
248
249
250
251
252
253
254
255
256
257
258
259
260
261
262
263
264
265
266
267
268
269
270
271
272
273
274
275
276
277
278
279
280
281
282
283
284
285
286
287
288
289
290
291
292
293
294
295
296
297
298
299
300
301
302
303
304
305
306
307
308
309
310
311
312
313
314
315
316
317
318
319
320
321
322
323
324
325
326
327
328
329
330
331
332
333
334
335
336
337
338
339
340
341
342
343
344
345
346
347
348
349
350
351
352
353
354
355
356
357
358
359
360
361
362
363
364
365
366
367
368
369
370
371
372
373
374
375
376
377
378
379
380
381
382
383
384
385
386
387
388
389
390
391
392
393
394
395
396
397
398
399
400
401
402
403
404
405
406
407
408
409
410
411
412
413
414
415
416
417
418
419
420
421
422
423
424
425
426
427
428
429
430
431
432
433
434
435
436
437
438
439
440
441
442
443
444
445
446
447
448
449
450
451
452
453
454
455
456
457
458
459
460
461
462
463
464
465
466
467
468
469
470
471
472
473
474
475
476
477
478
479
480
481
482
483
484
485
486
487
488
489
490
491
492
493
494
495
496
497
498
499
500
501
502
503
504
505
506
507
508
509
510
511
512
513
514
515
516
517
518
519
520
521
522
523
524
525
526
527
528
529
530
531
532
533
534
535
536
537
538
539
540
541
542
543
544
545
546
547
<!DOCTYPE html>
<html>
<head>
<title>Tidy text analysis</title>
<meta charset="utf-8">
<meta name="author" content="Devin Judge-Lord" />
<link rel="stylesheet" href="templates/xaringan-themer.css" type="text/css" />
</head>
<body>
<textarea id="source">
class: center, middle, inverse, title-slide
# Tidy text analysis
### Devin Judge-Lord
---
## 1. Counting
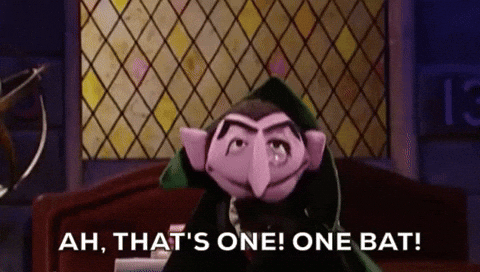
--
- text features `\(\in\)`\{all* words, some words, phrases, etc.\}
---
## 1. Counting
- text features `\(\in\)`\{all* words, some words, phrases, etc.\}
## 2. Matching

--
- Exactly* the same string ("regular expressions," text reuse)
---
## 1. Counting
- text features `\(\in\)`\{all* words, some words, phrases, etc.\}
## 2. Matching
- Exactly* the same string ("regular expressions," text reuse)
## 3. Classifying

---
## 1. Counting
- Text features `\(\in\)`\{all* words, some words, phrases, etc.\}
## 2. Matching
- Exactly* the same string ("regular expressions," text reuse)
- "Fuzzy" matching is exact matching a set of variants
## 3. Classifying
- Rules vs. probability
- Supervised vs. unsupervised
---
## Resources
- [Tidy text class by Andrew Heiss](https://datavizf18.classes.andrewheiss.com/class/11-class/)
- [`tidytext`](https://cran.r-project.org/web/packages/tidytext/tidytext.pdf) package
- Regular expressions with `stringr`--[cheasheet](http://edrub.in/CheatSheets/cheatSheetStringr.pdf)
- [Tidy text analysis](https://www.tidytextmining.com/) including
[topic modeling](https://www.tidytextmining.com/topicmodeling.html) and
[tidy() for Structural Topic Models](https://juliasilge.github.io/tidytext/reference/stm_tidiers.html) from the `stm` package. More [here](https://rdrr.io/cran/tidytext/man/stm_tidiers.html).
## Reading
- [Introduction to cluster analysis](https://eight2late.wordpress.com/2015/07/22/a-gentle-introduction-to-cluster-analysis-using-r/)
- Tidy Natural Language Processing with [`cleanNLP`](https://scholarship.richmond.edu/cgi/viewcontent.cgi?article=1195&context=mathcs-faculty-publications)
- [Text from audio](https://www.cambridge.org/core/journals/political-analysis/article/testing-the-validity-of-automatic-speech-recognition-for-political-text-analysis/E375085D96331A47E810C01AA6DB0A46)
- Here is an [ok blog post on visualizing qualitative data](https://depictdatastudio.com/how-to-visualize-qualitative-data/)--lmk if you find a better resource.
---
## Cool applications:
- [Gender tropes in film](https://pudding.cool/2017/08/screen-direction/)
- [Analysis of Trump's tweets confirms he writes only the (angrier) Android half](http://varianceexplained.org/r/trump-tweets/)
- [Every time Ford and Kavanaugh dodged a question, in one chart](https://www.vox.com/policy-and-politics/2018/9/28/17914308/kavanaugh-ford-question-dodge-hearing-chart)
---
class: inverse
# 1 Counting things in fancy ways.
--
With tidy text, counting words or phrases is simple:
- `unnest_tokens()` splits each response into tokens (by word by default, but we can also tokenize by phrases of length n, called n-grams).
- [optional] `anti_join(stop_words)` removes words that often have little meaning, like "a" and "the", called stop words. We can also do this with with `filter(!(word %in% stop_words$word))`
- `count()` how many times each word appears (`count(word)` is like `group_by(word) %>% summarize(n = n()) %>% ungroup()` )
---
### Word frequency
Responses to ANES question "What do you dislike about [Democrats/Republicans]?" (V161101, V161106)
```r
load(here("data/ANESdislikes.Rdata"))
d <- ANESdislikes
d[1,]
```
```
## # A tibble: 1 x 3
## V160001 question response
## <int> <chr> <chr>
## 1 300001 dislike_about… i see them trying take to much and give to the ho…
```
Tokenize by word
```r
words <- unnest_tokens(d, word, response)
head(words)
```
```
## # A tibble: 6 x 3
## V160001 question word
## <int> <chr> <chr>
## 1 300001 dislike_about_DEM i
## 2 300001 dislike_about_DEM see
## 3 300001 dislike_about_DEM them
## 4 300001 dislike_about_DEM trying
## 5 300001 dislike_about_DEM take
## 6 300001 dislike_about_DEM to
```
---
### Replace meaningless words
```r
# 1. anti-join() a data frame with a column of unwanted words called 'word'
words %<>% anti_join(stop_words)
# 2. Filter out strings we don't want separated with "|" (RegEx "or")
unwanted_words <- "people|just|dont|like|about|democrat.|republican.|party|[0-9]"
words %<>% filter(!str_detect(word, unwanted_words) )
```
### Count
```r
## Count the number of times each word occurs in each group
words %<>% group_by(question) %>% count(word)
head(words)
```
```
## # A tibble: 6 x 3
## # Groups: question [1]
## question word n
## <chr> <chr> <int>
## 1 dislike_about_DEM abandoning 1
## 2 dislike_about_DEM abaout 1
## 3 dislike_about_DEM abe 1
## 4 dislike_about_DEM abilities 1
## 5 dislike_about_DEM ability 5
## 6 dislike_about_DEM abomination 1
```
---
```r
top_n(words, 10) %>% ## Top 10 words in each group
ggplot( aes(x = reorder(word, n), y = n) ) +
geom_col() +
coord_flip() +
facet_wrap("question", scales = "free_y", strip.position="top") +
labs(x = "Word", y = "Count")
```
<img src="Figs/ANESfrequency-1.png" style="display: block; margin: auto;" />
---
```r
words %>%
filter(question == "dislike_about_GOP") %>%
with(wordcloud(word, n, max.words = 50))
```

---
Word clouds only show word frequency, and font size is hard to compare visually. Nevertheless, [they may be useful](https://www.vis4.net/blog/2015/01/when-its-ok-to-use-word-clouds/) if all you care about is frequency.
---
Tokenize by word pair ("bi-gram")
```r
## Name the new column d$bigrams ("ngrams" where n = 2)
bigrams <- unnest_tokens(d, bigram, response, token = "ngrams", n = 2)
head(bigrams)
```
```
## # A tibble: 6 x 3
## V160001 question bigram
## <int> <chr> <chr>
## 1 300001 dislike_about_DEM i see
## 2 300001 dislike_about_DEM see them
## 3 300001 dislike_about_DEM them trying
## 4 300001 dislike_about_DEM trying take
## 5 300001 dislike_about_DEM take to
## 6 300001 dislike_about_DEM to much
```
```r
## Count the number of times each bigram occurs in each group
bigrams %<>%
group_by(question) %>%
count(bigram)
```
---
```r
## Top 10 bigrams in each group
top_n(bigrams, 10) %>%
ggplot( aes(x = reorder(bigram, n), y = n) ) +
geom_col() +
coord_flip() +
facet_wrap("question", scales = "free_y", strip.position="top") +
labs(x = "Bigram", y = "Count")
```
<img src="Figs/ANESfrequency-b-1.png" style="display: block; margin: auto;" />
---
class: inverse
# 2 Matching
## Global alignment
(more counting than matching)
Used in: overall document similarity
## Local alignment
- Exact (`==`)
- Patterns (Regular expressions, `stringr`)
- Fuzzy patterns
Used in: text-reuse/plagiarism, web scraping, data cleaning
---
## Resources
Matching usually involve doing the same thing lots of times, so we use [functions](https://nicercode.github.io/guides/functions/)
- [`purrr`](https://www.rstudio.com/resources/cheatsheets/#purrr) offers tools to apply functions to data (instead of writing loops where we have to index everything)
Web scraping also requires manipulating HTML.
- [Khan Academy intro to HTML.](https://www.khanacademy.org/computing/computer-programming/html-css/intro-to-html/pt/html-basics)
- `rvest` offers tools for web scraping. Here is a [tutorial on Data Camp](https://www.datacamp.com/community/tutorials/r-web-scraping-rvest0)
Once we have scraped text, we need to clean it and extract the things we care about, both task for [RegEx]( https://www.rstudio.com/wp-content/uploads/2016/09/RegExCheatsheet.pdf)
- For tools to manipulate text, see the [stringr cheatsheet](https://www.rstudio.com/resources/cheatsheets/#stringr)
- Matt Denny has a [nice tutorial on cleaning text](http://www.mjdenny.com/Text_Processing_In_R.html)
---
## Global alignment
How similar are pairs of texts are overall (i.e., statistically)
1. Count things, usually word frequencies.
- Recall the [congressional letters example](https://judgelord.github.io/PS811/text-legislator-letters.html)
- The result is a high-dimension object. (If each word is a variable, we may have thousands of variables!)
2. Compare distributions
- Cosign similarity scores (pairwise similarity score)
- Dimensional scaling (reduce variation to one or two spatial dimensions)
---
<img src="Figs/seuss.jpg" width="500px" style="display: block; margin: auto;" /><img src="Figs/samedifferent.jpg" width="500px" style="display: block; margin: auto;" />
---
## Local alignment
 
---
#### Exact (`==`) vs. patterns (`str_detect("regular expression")`)
```r
strings <- c("Elmo", "Zoe", "Thing 1", "Thing 2",
"are your politics more like Sesame Street or Dr Seuss?")
strings == "Elmo" # Exact match?
```
```
## [1] TRUE FALSE FALSE FALSE FALSE
```
```r
strings == "Thing [0-9]" # Exact match?
```
```
## [1] FALSE FALSE FALSE FALSE FALSE
```
```r
str_detect(strings, "Thing [0-9]") # RegEx match?
```
```
## [1] FALSE FALSE TRUE TRUE FALSE
```
```r
str_extract_all(strings, "[A-Z][a-z]*", simplify = T) # stringr functions: str_....
```
```
## [,1] [,2] [,3] [,4]
## [1,] "Elmo" "" "" ""
## [2,] "Zoe" "" "" ""
## [3,] "Thing" "" "" ""
## [4,] "Thing" "" "" ""
## [5,] "Sesame" "Street" "Dr" "Seuss"
```
---
<img src="Figs/regex-animal.jpg" width="500px" style="display: block; margin: auto;" />
---
# Web scraping
`rvest`: `read_html()`, or
`rvest` + `httr`: `read_html( httr::GET() )`
99% of web scraping is finding the information we want and wrangling it into a data frame.
```r
html <- read_html("https://www.UN.org/") # The UN homepage
links <- html_nodes(html, "a") # "a" nodes are linked text
html_text(links)[2:7] # The text for links 2-7
```
```
## [1] "国际提高地雷意识和协助地雷行动日-4月4日"
## [2] "International Mine Awareness Day - 4 April"
## [3] "Journée internationale pour la sensibilisation au problème des mines et l’assistance à la lutte antimines - 4 avril"
## [4] "Международный день просвещения по вопросам минной опасности и помощи в деятельности, связанной с разминированием — 4 апреля"
## [5] "Día Internacional de información sobre el peligro de las minas y de asistencia para las actividades relativas a las minas, 4 de abril"
## [6] "اليوم الدولي للتوعية بخطر الألغام\r\n\r\n4 نيسان/أبريل\r\n"
```
[Example: A list of companies lobbying the U.S. Federal Energy Regulatory Commission](https://judgelord.github.io/correspondence/functions/DOE_FERC-company-scraper.html)
[Example: A table including the text of scanned pdf letters from Members of Congress](https://judgelord.github.io/correspondence/functions/DOE_FERC-scraper.html)
---
class: inverse center middle
## Text-reuse/plagerism
---
## Measuring similarity:
- Percent copied words, n-grams, sentences
- Percent aligned
- Global alignment (word frequencies) or relative topic proportions
## Measuring change across versions:
- Percent new words, n-grams, sentences
- Percent unaligned
- Change in global alignment (word frequencies) or topic proportions
---
### For example, changes in budget text and committee reports [[see more]()]:

---
### When similar words mean different things, edits matter:
2010: `"CLIMATE CHANGE The Committee continues to support the Administration's efforts to address climate change. Funding for its voluntary climate change programs are continued through this bill."`
2012: `"CLIMATE CHANGE This Committee remains skeptical of the Administration's efforts to re-package existing programs and to fund new ones in the name of climate change."`
---
### Edits may introduce new ideas in otherwise aligned texts.
The Smith-Waterman local alignment algorithm:
2017: `"FDA is implementing the Food Safety Modernization Act FSMA `and the XXXXXXXXX motivation XXXXXX for XXXXX the generic drug labeling rule `and regulation of tobacco products"`
2018: `"FDA is implementing the Food Safety Modernization Act FSMA `XXX XXX addressing XXXXXXXXX opioid XXX abuse XXX XXXXXXX XXXX XXXXXXXX XXXX `and regulation of tobacco products"`
---
## [Smith-Waterman](http://fridolin-linder.com/resources/2016/03/30/local-alignment.html)
Tracing a path through the matrix of the two documents, maximizing matches (technically, points for sequences of matches and mismatches).
<img src="Figs/smith-waterman.png" width="500px" style="display: block; margin: auto;" />
---
## N-gram window
Finding all exact matches of n words or more.
With a 4-gram window:
2010: "`CLIMATE CHANGE The Committee` continues to support `the Administration's efforts to` address climate change. Funding for its voluntary climate change programs are continued through this bill."`
2012: "`CLIMATE CHANGE This Committee` remains skeptical of `the Administration's efforts to` re-package existing programs and to fund new ones in the name of climate change."`
From this example: [Change and influence in budget texts]()
Another example: [Public comments on proposed regulations]()
---
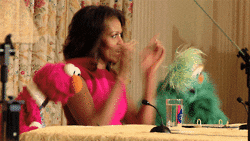
</textarea>
<style data-target="print-only">.remark-slide-container{display:block;}.remark-slide-scaler{box-shadow:none;}</style>
<script src="https://remarkjs.com/downloads/remark-latest.min.js"></script>
<script>var slideshow = remark.create({
"highlightStyle": "github",
"highlightLines": true,
"countIncrementalSlides": false
});
if (window.HTMLWidgets) slideshow.on('afterShowSlide', function (slide) {
window.dispatchEvent(new Event('resize'));
});
(function(d) {
var s = d.createElement("style"), r = d.querySelector(".remark-slide-scaler");
if (!r) return;
s.type = "text/css"; s.innerHTML = "@page {size: " + r.style.width + " " + r.style.height +"; }";
d.head.appendChild(s);
})(document);
(function(d) {
var el = d.getElementsByClassName("remark-slides-area");
if (!el) return;
var slide, slides = slideshow.getSlides(), els = el[0].children;
for (var i = 1; i < slides.length; i++) {
slide = slides[i];
if (slide.properties.continued === "true" || slide.properties.count === "false") {
els[i - 1].className += ' has-continuation';
}
}
var s = d.createElement("style");
s.type = "text/css"; s.innerHTML = "@media print { .has-continuation { display: none; } }";
d.head.appendChild(s);
})(document);
// delete the temporary CSS (for displaying all slides initially) when the user
// starts to view slides
(function() {
var deleted = false;
slideshow.on('beforeShowSlide', function(slide) {
if (deleted) return;
var sheets = document.styleSheets, node;
for (var i = 0; i < sheets.length; i++) {
node = sheets[i].ownerNode;
if (node.dataset["target"] !== "print-only") continue;
node.parentNode.removeChild(node);
}
deleted = true;
});
})();</script>
<script>
(function() {
var links = document.getElementsByTagName('a');
for (var i = 0; i < links.length; i++) {
if (/^(https?:)?\/\//.test(links[i].getAttribute('href'))) {
links[i].target = '_blank';
}
}
})();
</script>
<script>
(function() {
var i, text, code, codes = document.getElementsByTagName('code');
for (i = 0; i < codes.length;) {
code = codes[i];
if (code.parentNode.tagName !== 'PRE' && code.childElementCount === 0) {
text = code.textContent;
if (/^\\\((.|\s)+\\\)$/.test(text) || /^\\\[(.|\s)+\\\]$/.test(text) ||
/^\$\$(.|\s)+\$\$$/.test(text) ||
/^\\begin\{([^}]+)\}(.|\s)+\\end\{[^}]+\}$/.test(text)) {
code.outerHTML = code.innerHTML; // remove <code></code>
continue;
}
}
i++;
}
})();
</script>
<!-- dynamically load mathjax for compatibility with self-contained -->
<script>
(function () {
var script = document.createElement('script');
script.type = 'text/javascript';
script.src = 'https://cdn.bootcss.com/mathjax/2.7.1/MathJax.js?config=TeX-AMS_HTML';
if (location.protocol !== 'file:' && /^https?:/.test(script.src))
script.src = script.src.replace(/^https?:/, '');
document.getElementsByTagName('head')[0].appendChild(script);
})();
</script>
</body>
</html>