-
Notifications
You must be signed in to change notification settings - Fork 12
New issue
Have a question about this project? Sign up for a free GitHub account to open an issue and contact its maintainers and the community.
By clicking “Sign up for GitHub”, you agree to our terms of service and privacy statement. We’ll occasionally send you account related emails.
Already on GitHub? Sign in to your account
关于Generalization to large models章节中的参数问题 #2
Comments
@MaybeShewill-CV 你好,你跑一张图像多长时间?准确率怎样? |
@Soulempty tensorrt 上不计算显存拷贝的开销能到105fps左右 不过我是gtx 1070显卡 性能比较差:) |
@Soulempty ok 加你了 |
Hi, @MaybeShewill-CV Can you show the code? I can't reduce my loss on my code which is followed the author thinking. And I don't know what's wrong with my code. It's too slow to decrease the loss. Here are my code: StemBlock# StemBlock
class StemBlock(nn.Module):
def __init__(self, in_ch=3, out_ch=16):
# in_ch: default is 3.
# out_ch: default is 16.
super(StemBlock, self).__init__()
self.conv1 = nn.Conv2d(in_ch, out_ch, kernel_size=3, stride=2, padding=1)
self.conv2 = nn.Conv2d(out_ch, out_ch//2, kernel_size=1, stride=1)
self.conv3 = nn.Conv2d(out_ch//2, out_ch, kernel_size=3, stride=2, padding=1)
self.conv4 = nn.Conv2d(out_ch * 2, out_ch, kernel_size=3, stride=1, padding=1)
self.maxPooling = nn.MaxPool2d(kernel_size=3, stride=2, padding=1)
self.bn1 = nn.BatchNorm2d(num_features=out_ch)
self.bn2 = nn.BatchNorm2d(num_features=out_ch//2)
self.relu = nn.ReLU()
def forward(self, x):
x = self.conv1(x)
# x1
x1 = self.conv2(x)
x1 = self.relu(self.bn2(x1))
x1 = self.conv3(x1)
# [H/4, W/4, C]
x1 = self.relu(self.bn1(x1))
# x2
x2 = self.relu(self.bn1(x))
# [H/4, W/4, C]
x2 = self.maxPooling(x2)
x = torch.cat((x1, x2), 1)
x = self.conv4(x)
x = self.relu(self.bn1(x))
return x ContextEmbeddingBlock:class ContextEmbeddingBlock(nn.Module):
def __init__(self, in_ch=128, out_ch=128, stride=1):
super(ContextEmbeddingBlock, self).__init__()
self.gapPooling = nn.AdaptiveAvgPool2d((1,1))
self.bn = nn.BatchNorm2d(num_features=in_ch)
self.conv1 = nn.Conv2d(in_ch, out_ch, kernel_size=1, stride=1)
self.relu = nn.ReLU()
self.conv2 = nn.Conv2d(in_ch, out_ch, kernel_size=3, stride=1, padding=1)
def forward(self, x):
x1 = self.gapPooling(x)
# [None, C, 1, 1]
if not x1.size()[0] == 1:
x1 = self.bn(x1)
x1 = self.conv1(x1)
if not x1.size()[0] == 1:
x1 = self.relu(self.bn(x1))
else:
x1 = self.relu(x1)
x = torch.add(x1, x)
x = self.conv2(x)
return x DWConv:class DWConv(nn.Module):
def __init__(self, in_ch, out_ch, stride=1):
super(DWConv, self).__init__()
self.depth_conv = nn.Conv2d(in_ch, in_ch,
kernel_size=3,
stride=stride,
padding=1,
groups=in_ch)
self.point_conv = nn.Conv2d(in_ch, out_ch,
kernel_size=1,
stride=1,
groups=1)
def forward(self, x):
x = self.depth_conv(x)
x = self.point_conv(x)
return x GatherAndExpansionLayer:class GatherAndExpansionLayer(nn.Module):
def __init__(self, in_ch, out_ch, stride=1):
super(GatherAndExpansionLayer, self).__init__()
error = "GatherAndExpansionLayer's stride only support 1 or 2."
assert stride in [1, 2], error
self.stride = stride
if self.stride == 2:
self.conv2_1 = nn.Conv2d(in_ch, in_ch, kernel_size=3, stride=1, padding=1)
self.conv2_2 = DWConv(in_ch, 6 * in_ch, stride=2)
self.conv2_3 = DWConv(6 * in_ch, 6 * in_ch, stride=1)
self.conv2_4 = nn.Conv2d(6 * in_ch, out_ch, kernel_size=1)
self.conv2_5 = DWConv(in_ch, out_ch, stride=2)
self.conv2_6 = nn.Conv2d(out_ch, out_ch, kernel_size=1, stride=1)
else:
self.conv1_1 = nn.Conv2d(in_ch, in_ch, kernel_size=3, stride=self.stride, padding=1)
self.conv1_2 = DWConv(in_ch, 6 * in_ch, stride=self.stride)
self.conv1_3 = nn.Conv2d(6 * in_ch, out_ch, kernel_size=1, stride=self.stride)
self.bn1 = nn.BatchNorm2d(num_features=in_ch)
self.bn2 = nn.BatchNorm2d(num_features=in_ch * 6)
self.bn3 = nn.BatchNorm2d(num_features=out_ch)
self.relu = nn.ReLU()
def forward(self, x):
if self.stride == 2:
x1 = self.conv2_1(x)
x1 = self.relu(self.bn1(x1))
x1 = self.conv2_2(x1)
x1 = self.bn2(x1)
x1 = self.conv2_3(x1)
x1 = self.bn2(x1)
x1 = self.conv2_4(x1)
x1 = self.bn3(x1)
x2 = self.conv2_5(x)
x2 = self.bn3(x2)
x2 = self.conv2_6(x2)
x2 = self.bn3(x2)
else:
x1 = self.conv1_1(x)
x1 = self.relu(self.bn1(x1))
x1 = self.conv1_2(x1)
x1 = self.bn2(x1)
x1 = self.conv1_3(x1)
x1 = self.bn3(x1)
x2 = x
x = torch.add(x1, x2)
x = self.relu(x)
return x DetailBranch:class DetailBranch(nn.Module):
def __init__(self):
super(DetailBranch, self).__init__()
self.conv1 = nn.Conv2d(3, 64, kernel_size=3, stride=2, padding=1)
self.conv2 = nn.Conv2d(64, 64, kernel_size=3, stride=1, padding=1)
self.conv3 = nn.Conv2d(64, 64, kernel_size=3, stride=2, padding=1)
self.conv4 = nn.Conv2d(64, 128, kernel_size=3, stride=2, padding=1)
self.conv5 = nn.Conv2d(128, 128, kernel_size=3, stride=1, padding=1)
self.bn1 = nn.BatchNorm2d(num_features=64)
self.bn2 = nn.BatchNorm2d(num_features=128)
self.relu = nn.ReLU()
def forward(self, x):
# S1
x = self.relu(self.bn1(self.conv1(x)))
x = self.relu(self.bn1(self.conv2(x)))
# S2
x = self.relu(self.bn1(self.conv3(x)))
x = self.relu(self.bn1(self.conv2(x)))
x = self.relu(self.bn1(self.conv2(x)))
# S3
x = self.relu(self.bn2(self.conv4(x)))
x = self.relu(self.bn2(self.conv5(x)))
x = self.relu(self.bn2(self.conv5(x)))
return x SemanticBranch:class SemanticBranch(nn.Module):
def __init__(self, is_train=False):
super(SemanticBranch, self).__init__()
self.is_train = is_train
# S1 and S2
self.stem = StemBlock(in_ch=3, out_ch=16)
# S3
self.ge1 = GatherAndExpansionLayer(in_ch=16, out_ch=32, stride=2)
self.ge2 = GatherAndExpansionLayer(in_ch=32, out_ch=32, stride=1)
# S4
self.ge3 = GatherAndExpansionLayer(in_ch=32, out_ch=64, stride=2)
self.ge4 = GatherAndExpansionLayer(in_ch=64, out_ch=64, stride=1)
# S5
self.ge5 = GatherAndExpansionLayer(in_ch=64, out_ch=128, stride=2)
self.ge6 = GatherAndExpansionLayer(in_ch=128, out_ch=128, stride=1)
self.ce = ContextEmbeddingBlock(in_ch=128, out_ch=128, stride=1)
def forward(self, x):
# S1 and S2
out1 = self.stem(x)
# out1: [None, 16, 128, 256]
# S3
x = self.ge1(out1)
out2 = self.ge2(x)
# out2: [None, 32, 64, 128]
# S4
x = self.ge3(out2)
out3 = self.ge4(x)
# out3: [None, 64, 32, 64]
# S5
x = self.ge5(out3)
x = self.ge6(x)
x = self.ge6(x)
out4 = self.ge6(x)
# out4: [None, 128, 16, 32]
# x: [None, 128, 16, 32]
x = self.ce(out4)
if self.is_train:
return x, out1, out2, out3, out4
else:
return x SegHeadBooster:class SegHeadBooster(nn.Module):
def __init__(self, in_ch, t_ch, out_ch, size):
super(SegHeadBooster, self).__init__()
self.conv1 = nn.Conv2d(in_ch, t_ch, kernel_size=3, stride=1, padding=1)
self.conv2 = nn.Conv2d(t_ch, out_ch, kernel_size=1, stride=1, bias=False)
self.bn = nn.BatchNorm2d(num_features=t_ch)
self.relu = nn.ReLU()
self.size = size
def forward(self, x):
x = self.conv1(x)
x = self.relu(self.bn(x))
x = self.conv2(x)
x = nn.functional.interpolate(x, size=self.size, mode='bilinear')
return x BiseNet_V2:class BiseNet_V2(nn.Module):
def __init__(self, num_classes=19, is_train=False):
super(BiseNet_V2, self).__init__()
self.is_train = is_train
# Common
self.dw = DWConv(in_ch=128, out_ch=128, stride=1)
self.bn = nn.BatchNorm2d(num_features=128)
self.conv1_1 = nn.Conv2d(128, 128, kernel_size=1, stride=1)
self.sigmoid = nn.Sigmoid()
# Detail Branch
self.detailBranch = DetailBranch()
self.conv3_3_2 = nn.Conv2d(128, 128, kernel_size=3, stride=2, padding=1)
self.avgPooling = nn.AvgPool2d(kernel_size=3, stride=2, padding=1)
# Semantic Branch
self.semanticBranch = SemanticBranch(is_train=self.is_train)
self.conv3_3_1 = nn.Conv2d(128, 128, kernel_size=3, stride=1, padding=1)
# Seg Head
size = (512, 1024)
self.segHead0 = SegHeadBooster(in_ch=128, t_ch=64, out_ch=num_classes, size=size)
self.segHead1 = SegHeadBooster(in_ch=16, t_ch=64, out_ch=num_classes, size=size)
self.segHead2 = SegHeadBooster(in_ch=32, t_ch=64, out_ch=num_classes, size=size)
self.segHead3 = SegHeadBooster(in_ch=64, t_ch=64, out_ch=num_classes, size=size)
self.segHead4 = SegHeadBooster(in_ch=128, t_ch=64, out_ch=num_classes, size=size)
# init weight
self.init_weight()
def forward(self, x):
# Detail Branch
dx = self.detailBranch(x)
dx1 = self.bn(self.dw(dx))
dx1 = self.conv1_1(dx1)
dx2 = self.bn(self.conv3_3_2(dx))
dx2 = self.avgPooling(dx2)
# Semantic Branch
if self.is_train:
sx, out1, out2, out3, out4 = self.semanticBranch(x)
out1 = self.segHead1(out1)
out2 = self.segHead2(out2)
out3 = self.segHead3(out3)
out4 = self.segHead4(out4)
else:
sx = self.semanticBranch(x)
sx1 = self.bn(self.dw(sx))
sx1 = self.sigmoid(self.conv1_1(sx1))
sx2 = self.bn(self.conv3_3_1(sx))
sx2 = nn.functional.interpolate(sx2, scale_factor=4, mode='bilinear')
sx2 = self.sigmoid(sx2)
# Aggregation Layer: element-wise product
d_out = torch.mul(dx1, sx2)
s_out = torch.mul(dx2, sx1)
s_out_ = nn.functional.interpolate(s_out, scale_factor=4, mode='bilinear')
out = d_out.add(s_out_)
# out: [None, 128, 64, 128]
out0 = self.bn(self.conv3_3_1(out))
out0 = self.segHead0(out0)
if self.is_train:
return out0, out1, out2, out3, out4
else:
return out0
def init_weight(self):
# Kaiming Normal
for ly in self.children():
if isinstance(ly, nn.Conv2d):
nn.init.kaiming_normal_(ly.weight, a=1)
if not ly.bias is None: nn.init.constant_(ly.bias, 0) |
@MikoyChinese I will release the code when prepared. I'm looking forward to the author's official implementation:) |
@MikoyChinese I have released my implementation. |
Thanks for your great work. |
@MikoyChinese Welcome. Still look forward origin implementation:) |
@ncuxiaogang 我之前的理解也是d指代module repeat的次数 不知道是不是原作者想表达的意思 哈哈 |
看了下你的代码实现,想请教下: |
@ncuxiaogang 1.Ct是我自己设置的 不知道是不是符合原作者的意思 2.CoinCheung 实现的是bisenetv1不是bisenetv2哈 :) |
里面有个BisenetV2文件夹,你可以进去看下 |
@ncuxiaogang 好的 我看看 有段时间没看了 可能更新过了吧 |
首先感谢大佬 @ycszen 的工作:)
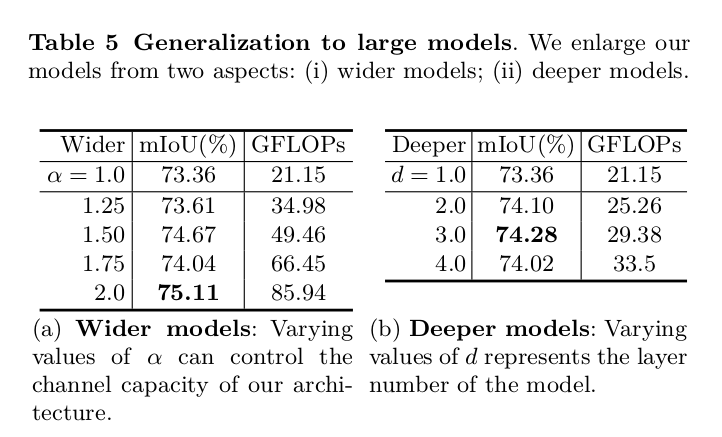
文章中有些我觉得写的不是很清楚的地方想问一下,关于generalization to large models章节中提到的两个参数α和d。
关于α,我觉得指的是进入segmentation heads模块之前的channel expansion倍数,如下图所示
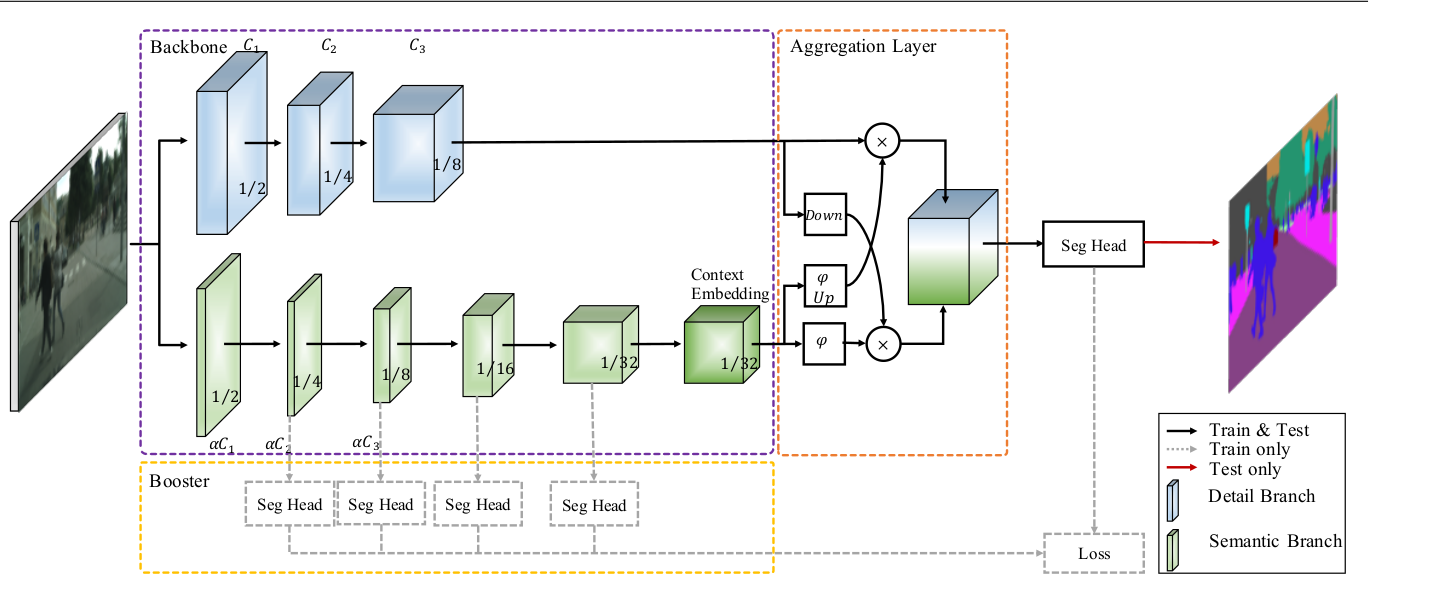
那么这个d参数指的是什么呢,文章中说d参数控制的是模型的深度,那么这个d是指代上文中的什么参数呢,是module repeat的次数吗.
还有点疑惑就是table5下方的描述α控制模型的channel capacity而d控制layer nums.然后下文中说α是width multiplier,d是depth multiplier。请问这个地方上下文的表述是否一致。
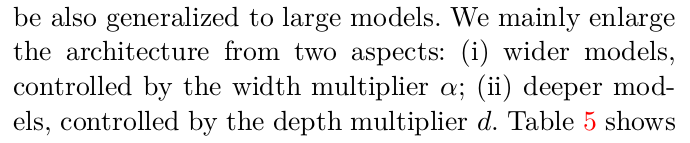
这两个参数该如何理解更为准确呢。还请大佬有时间不吝赐教 :)
By the way 初步实现了下 确实很快:)
The text was updated successfully, but these errors were encountered: